The pharmaceutical industry constantly faces time and cost pressure to advance drugs that bring long-lasting impacts to society. Drug discovery is instrumental in supporting the broader healthcare ecosystem, which calls for a timely transformational intervention. Generative AI, which made an impressive debut, is positioned to positively transform and optimize pharmaceutical workflow.
Industry leaders are buoyed by the prospect of generative AI in the pharmaceutical industry. According to a market study, the global market value for generative AI pharmaceutical use cases is projected to hit USD 2.2 billion by 2032, a 31.2% CAGR from USD 159.9 million in 2022. As product managers in your respective organization, the pharmaceutical AI market looks promising.
Yet, generative AI is still an evolving technology, with risks and challenges that might affect the positive outcomes it offers. As Uptech’s co-founder, I’ve been leading our tech team in integrating generative AI into new and existing apps. For example, we built Dyvo AI, an app that uses deep learning algorithms to generate social media avatars, studio-like headshots, and ecommerce photoshoots.
In this article, I’ll explain how generative AI benefits pharmaceutical practices, its challenges, and ways to mitigate concerns when developing AI solutions.

Generative AI in the Pharmaceutical Industry
The global pandemic has spurred pharmaceutical giants to accelerate vaccine development at an unprecedented pace. Processes that took years or decades were completed in months as drug researchers raced to save lives amidst uncertainties. It also drew attention to the intricate process of formulating, validating, and testing new drugs before pharmaceutical companies can safely release them to the public.
Generative artificial intelligence (AI) is a machine learning technology capable of generating new, unique content by drawing from knowledge it learns from massive datasets it trained on. Drawing from recent events, pharmaceutical leaders have demonstrated a keen interest in integrating AI to accelerate drug discovery and other areas.
Typically, drug discovery involves a tedious process called retrosynthesis, where chemists identify the desired molecule compound and work backward to produce it. The task includes examining different chemical reactions, hoping one would lead to the formulation of safe and effective drugs. Such endeavors could take years or decades without assurance that investments will translate into success.
With generative AI, pharmaceutical companies seek to reduce the timeline by automating and accelerating part of the processes. For example, machine learning engineers introduced G2Retro, an alternative retrosynthesis method that applies a deep learning model to predict optimal chemical reactions from a vast range of molecule compounds.
By bringing generative AI to the fold, pharmaceutical companies not only reduce drug development timelines but also streamline marketing, logistics, customer support, and other operations.
Generative AI Use Cases in the Pharmaceutical Industry
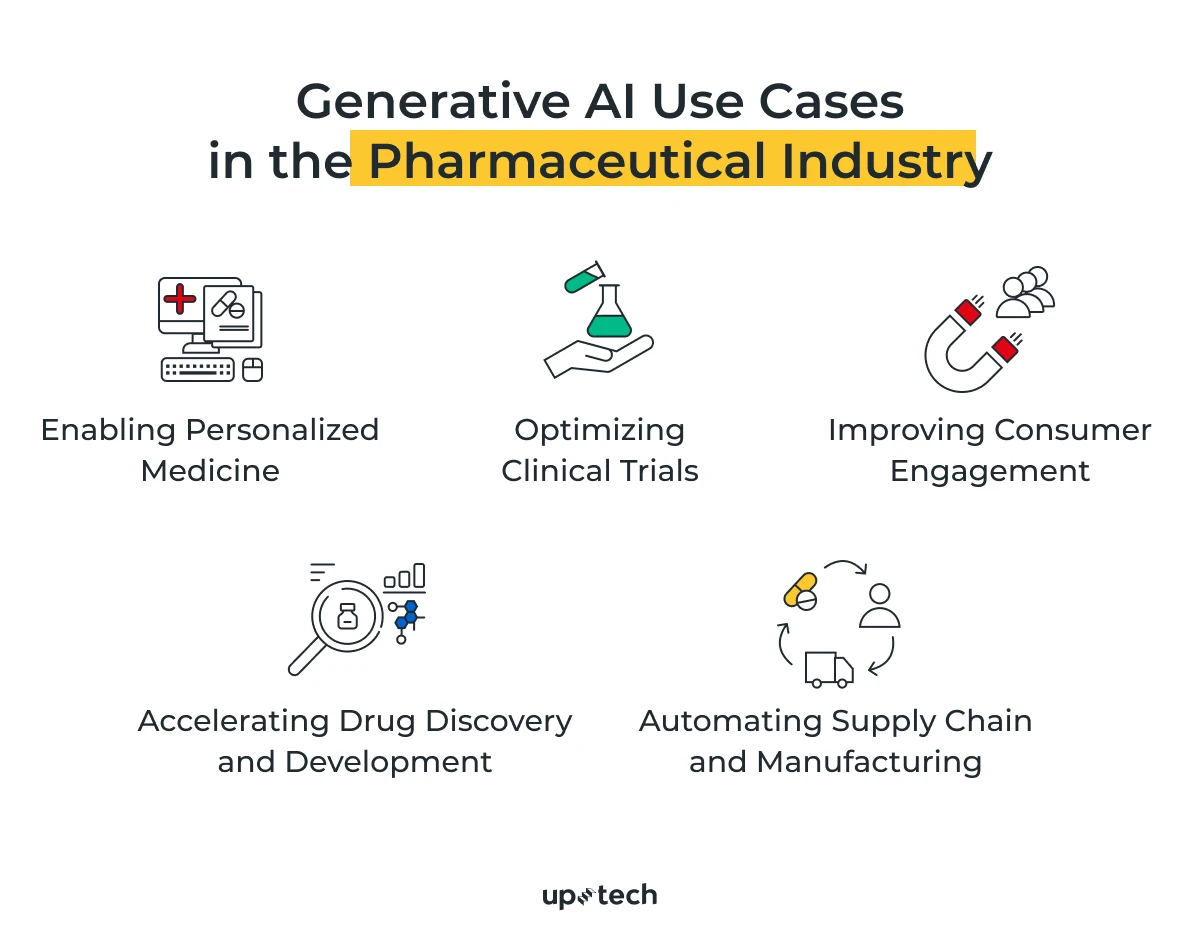
Let’s explore how generative AI can add value to pharmaceutical companies.
Accelerating Drug Discovery and Development
Generative AI can accelerate drug discovery by analyzing molecule properties, projecting compound models, predicting combination outcomes, and forecasting potential adverse effects of new drugs. Besides shortening the time to market, generative AI also reduces the exhortative cost that drug development usually incurs. For example, Insilico Medicine leveraged a generative AI platform to discover a novel drug for treating pulmonary diseases. By using AI, the company paid only 10% of the estimated cost of $400 million.
Enabling Personalized Medicine
Generative AI can tailor and deliver patients' medical needs without excessive human intervention. Pharmaceutical companies can integrate AI to analyze electronic health records, lab reports, and patient interactions to determine the appropriate drugs they need. The medical summaries AI generates will augment physicians' efforts to deliver better patient outcomes. For example, doctors use generative AI to assess if a patient is potentially allergic to specific drugs before providing a prescription.
Optimizing Clinical Trials
Clinical trials require careful planning as they impact the candidate’s safety and drug research outcome. Generative AI can influence the trial’s success by analyzing medical literature, patient data, hypotheses, and other relevant information researchers may overlook. Pharmaceutical companies can use AI predictions to estimate costs, risks, and probable results before proceeding with clinical trials.
Automating Supply Chain and Manufacturing `
Generative AI can analyze market trends and predict drug demands in regional markets. It helps pharmaceutical firms improve logistic and infrastructural workflows by generating data-driven insights to ensure supply availability and mitigate cost fluctuations. Likewise, integrating generative AI with drug manufacturing facilities enables better inventory management, waste reduction, and process efficiency.
Improving Consumer Engagement
Pharmaceutical firms can improve their branding strategy and customer engagement by using AI virtual chatbots. By training the chatbots with customer records, historical transactions, and drug catalogs, pharmaceutical companies can promptly address queries, provide 24/7 support, and send personalized marketing messages to their customers. Patients can also seek information about potential drug interactions and side effects without switching to different platforms.
Challenges of Generative AI in Pharma Industry
Generative AI in healthcare will reshape the pharmaceutical sector, bringing efficiency, automation, and sustainability for manufacturers and customers alike. However, there are challenges that you must be mindful of before introducing AI products into a strictly regulated industry.
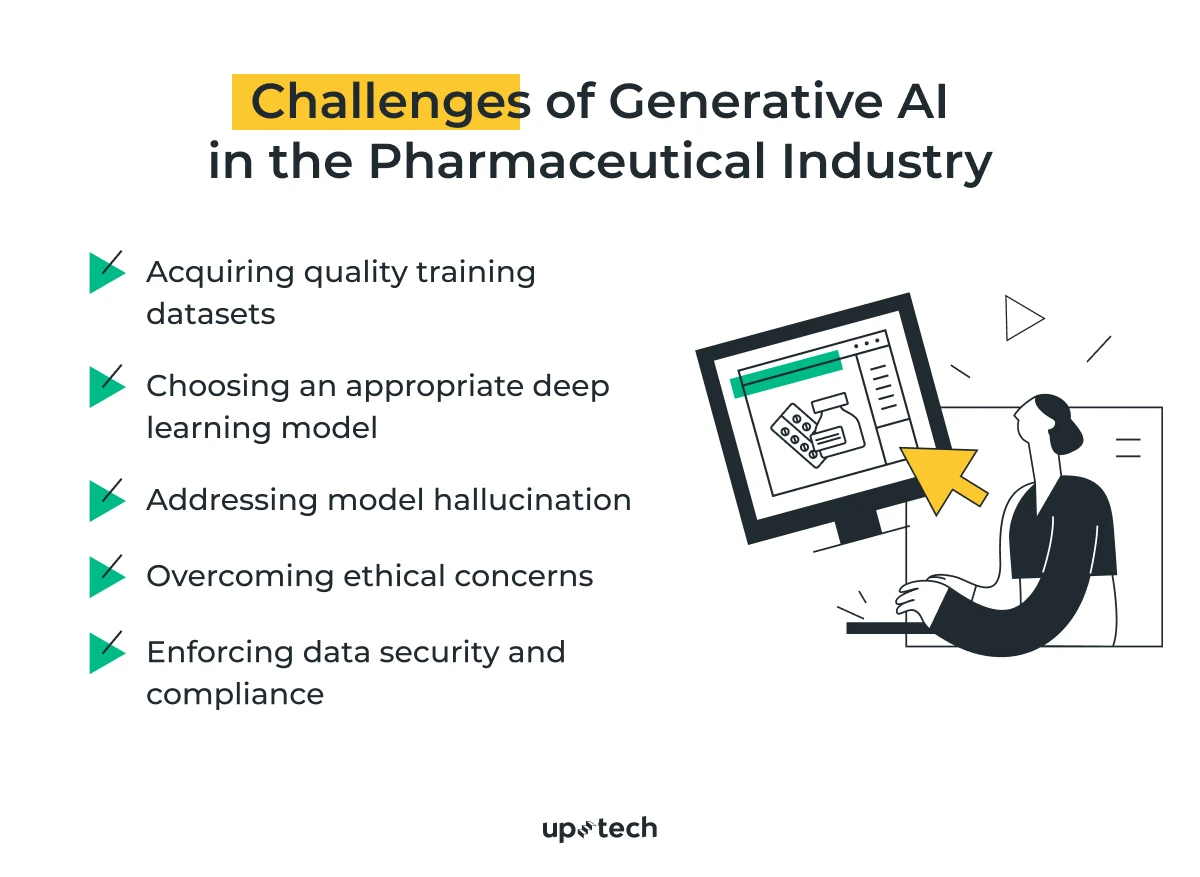
Acquiring quality training datasets
Generative AI is powered by deep learning models that require training and fine-tuning before they can function optimally. Training these models requires massive datasets curated from reliable sources. If you feed AI models with low-quality datasets, they will perform sub-optimally in real-life applications. Here, the challenge lies in compiling a sizeable dataset sufficient to train an accurate, reliable, and consistent AI system.
Unlike general tasks like copywriting, pharmaceutical use cases involve highly specialized knowledge not commonly accessible to the public. Comparatively, it’s harder to curate diverse training samples to train a pharma AI model than to develop a generic AI chatbot.
Choosing an appropriate deep learning model
As generative AI’s potential became apparent, many leading AI providers have joined the race to introduce their own deep learning models. Each model differs in accuracy, truthfulness, summarization, and other metrics that affect their suitability in medical use cases. To choose a generative AI model for pharmaceutical applications, you must compare numerous algorithms and assess if they fit your product requirements.
For example, the convolutional neural network is more suitable for medical image analysis and doesn’t fit well for drug discovery. Instead, models like generative adversarial networks and transformers are a better fit for analyzing medical literature and predicting drug formulations.
Addressing model hallucination
Model hallucination is a phenomenon where the machine learning algorithm produces plausible but incorrect results. In pharmaceutical practices, such inaccuracies can influence decisions when formulating drugs, dispensing them, and other customer-related interactions. To complicate the matter, model hallucination is hard to spot unless supervised by attentive, medically trained experts. Therefore, pharmaceutical companies must apply safeguards to prevent such inaccuracies from affecting drug production and patient’s well-being.
Overcoming ethical concerns
Deep learning models that generative AI uses lack explainability and visibility. They are made of dozens of hidden layers that make autonomous inferences based on previous input. When applied, pharmaceutical companies might find it challenging to explain the model's decisions. Moreover, some models may exhibit biased, offensive, or downright harmful behaviors to brands and customers.
For example, an AI model could inaccurately project drug demands based on economic factors that favor high-income earners. This happens when data scarcity results in training samples that don’t represent pharmaceutical customers fairly. Trained with a biased dataset, the AI model will generate unfair recommendations.
Enforcing data security and compliance
Pharma companies are obliged, by ethics and laws, to safeguard patients’ privacy. Training and deploying generative AI models require curating and managing large volumes of data on the cloud. Rightfully, users are concerned about data breaches and misappropriation that will result in personal theft and other negative implications. Several regulations, such as HIPAA, HITECH, and GPDR, require pharmaceutical firms to apply robust data security measures in their respective regions.
Tips for Using Generative AI in the Pharmaceutical Industry
Understandably, pharmaceutical leaders may hesitate to implement generative AI at scale with the looming challenges I mentioned. Drawing from our experience developing AI-powered apps, I share several tips to build secure, functional, and responsible pharmaceutical AI solutions below.
- Focus your effort on curating diverse and quality datasets. Collaborate with physicians, medical researchers, and pharmaceutical researchers to assess and prepare the training datasets.
- Apply security measures to ensure data integrity and security along the machine learning pipeline. Strictly comply with HIPAA and other regulations to safeguard customer’s privacy.
- I recommend using a pre-trained model because of the enormous cost and time to train one from scratch. However, it’s imperative to evaluate pre-trained models before fine-tuning them with pharmaceutical datasets. Pre-trained models vary in performance and may inherit bias and other performance issues from the dataset they train on.
- Generative AI may not always produce accurate arithmetic results. If you use deep learning models for mathematical calculations, such as projecting supply chain expenses, ensure that human reviewers approve them.
- Continuously train the AI model with anonymized data from real-time usage to improve its accuracy and overall performance.

How can Uptech help?
Navigating the technical complexities and regulatory requirements that pharmaceutical AI demands proves challenging for many startups, businesses, and enterprises.
Uptech, with years of app development experience, is a forerunner in generative AI technologies and we offer top-quality custom AI development services. Despite being an evolving technology, our team has successfully implemented deep learning models in new and existing digital solutions.
For example, we integrated an AI recommendation system with Plai, which helps HR managers streamline employee performance reviews. With generative AI, managers can use the generated recommendations to devise career growth plans tailored to each employee.
Another notable work is Hamlet, an AI-powered summarizer leveraging deep learning models to extract key information from documents and compact them into human-understandable text.

Besides AI, our app team is equally adept in developing solutions for the healthcare space. One of our works, a mental health app, serves the US market and complies with regulatory requirements.

We took pride in helping our clients get to the market quickly and affordably. For example, we can release a proof of concept within 30 days without exceeding $20k. Of course, this depends on the product’s complexity, which varies among clients.
Summary
Generative AI promises drug discovery acceleration, clinical trial optimization, cost reduction, and more in the pharma industry. By training deep learning models with pharmaceutical datasets, medical companies can bridge the supply gap and ensure customers can access appropriate prescriptions.
Still, several challenges could hinder industry-wide AI implementation. I’ve discussed quality datasets, model selection, ethical concerns, and other issues that require attention. I’ve also shown how pharmaceutical providers should mitigate such concerns when developing generative AI solutions.
Drop us a message to learn how we can aid you and avoid known obstacles in pharmaceutical AI.
FAQs
How is generative AI used in pharmaceutical industry?
Generative AI is a machine learning technology capable of generating new, unique content by drawing from knowledge it learns from massive datasets it trained on. Drawing from recent events, pharmaceutical leaders have demonstrated a keen interest in integrating AI to accelerate drug discovery and other areas.
By bringing generative AI to the fold, pharmaceutical companies not only reduce drug development timelines but also streamline marketing, logistics, customer support, and other operations.
What are examples of generative AI in pharma?
With generative AI, pharmaceutical companies seek to reduce the timeline by automating and accelerating part of the processes. For example, machine learning engineers introduced G2Retro, an alternative retrosynthesis method that applies a deep learning model to predict optimal chemical reactions from a vast range of molecule compounds.
What is generative AI in pharmaceutical industry?
Generative AI is a machine learning technology capable of generating new, unique content by drawing from knowledge it learns from massive datasets it trained on. Drawing from recent events, pharmaceutical leaders have demonstrated a keen interest in integrating AI to accelerate drug discovery and other areas.