ChatGPT brought generative AI, a next-generational machine learning capability, to the public. It sends scores of startups and businesses searching for how to build AI applications of similar caliber. Specifically, companies demonstrate significant interest in applying generative AI at work. In the US, 37% of professionals in the marketing and advertising sector have used generative AI in their jobs.
Understandably, you'll want to dive deeper into generative AI, particularly how it works and ways it could empower your business. As the co-founder and tech lead of Uptech, I've seen the AI space unfold over the years. We've built AI-powered apps such as Dyvo.ai and AI assistant for our HR performance tool - Plai, which helps our clients solve real-world problems more efficiently.
In this article, I'll share my thoughts on generative AI and how you can integrate state-of-the-art technology into your digital products.
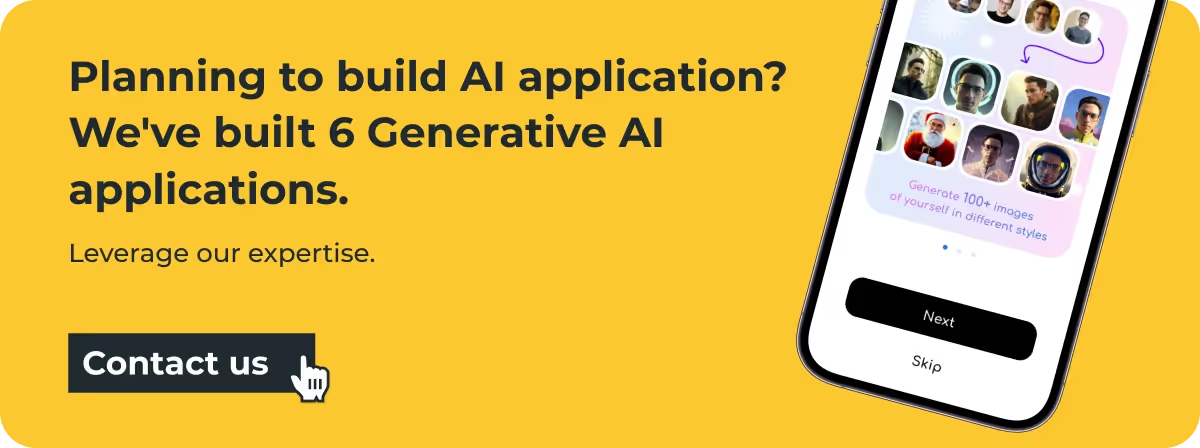
What is Generative AI
Generative AI is a specific discipline in machine learning that allows computers to create new and exciting content automatically. With generative AI, the software can generate unique text, images, videos, or audio after being trained with relevant datasets. During the training, the AI algorithm learns specific patterns from the provided samples, remembers them, and uses the retained memories to create new outputs in a similar style.
At a glance, generative AI sounds simple, but it's not. Historically, works on generative AI date back to the 1950s, when researchers explored the foundational principles of artificial intelligence. Back then, they work with simple neural networks or rule-based models to mimic how humans think and make decisions.
Over the decades, data scientists have made tremendous progress, particularly in developing deep-learning neural networks. Some early models, Bayesian and Markov, are helpful in computer vision and robotics systems. But the birth of modern generative AI systems was rooted in 2014 when researchers unveiled the Generative Adversarial Network (GAN). And accelerated in 2017 with the introduction of Transformer architecture.
Today, generative AI tools like ChatGPT, Midjourney, Dall-E, and Bard harness the power of various neural networks, including diffusion networks, GAN, transformers, and variational autoencoders, to produce new, human-like content.
- A diffusion network trains with image samples by gradually adding noise to the source and backtracking the steps to generate realistic data representations.
- GAN is a deep-learning model that generates and checks its output to ensure they pass off as genuine data.
- Transformer is a neural network that allows a large language model to train with more input simultaneously. It introduces the attention concept, allowing the model to determine the importance of specific words in a sentence.
- Variational autoencoder derives and stores a probabilistic representation of the training samples, which it then uses as a reference to create original outputs.
A Glance at the Generative AI market
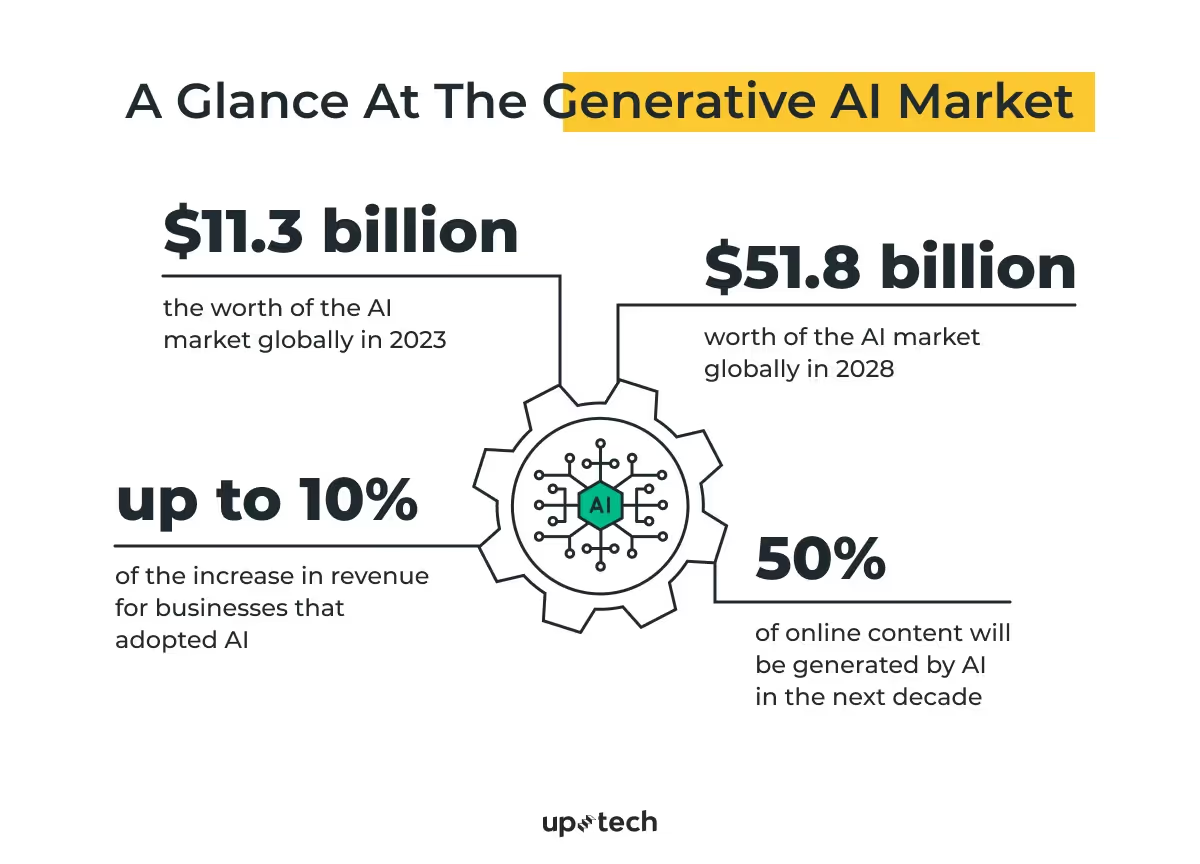
Financially, businesses have a huge incentive to embark on the generative AI train, whether as a provider or users. Studies revealed that the AI market is worth $11.3 billion globally. The figure is predicted to grow 35.6% over the next few years to $51.8 billion in 2028. Moreover, businesses that adopted AI in their workflow have demonstrated up to a 10% increase in revenue.
Even as I pen my thoughts, the generative AI space continues to evolve rapidly. New large language models are being released and trained with billions of parameters. Ordinary users are amazed by the capabilities of AI tools like MidJourney in generating vivid, realistic images. Likewise, marketers turn to linguistic models like GPT-4 to create engaging copies.
Here are some interesting insights on where the generative AI market is heading.
- The Covid pandemic has fueled the adoption of generative AI solutions, with major players like AWS, Google, and Microsoft taking the lead.
- Both diffusion and transformer neural networks continue to drive emerging AI systems.
- AI-generated content, including text and images, will make up 50% of online content in the next decade.
- With companies like OpenAI, Nvidia, and Google at the fore, the US continues to lead the generative AI market.
- Wide adoption is expected in various industries, including AR/VR, healthcare, retail, cloud computing, and media.
All in all, there is optimism in the air about how one of the most exciting technologies in modern times will stamp its mark on our life.
What are the benefits of generative AI?
Beneath the buzz brought upon by ChatGPT and its likes, there are real benefits of these advanced machine learning models to real-life applications. I share several notable examples below.

- Improved efficiency. Generative AI immediately impacts business productivity by allowing professionals to perform repetitive tasks quickly. For example, AI software can help marketers create a marketing plan in seconds, a process normally taking hours. This way, you can allocate your resources to other creative or people-intense tasks.
- Automation-friendly. Many businesses are streamlining their workflow to enable better employee collaboration. Generative AI automation allows companies to access robust AI capabilities and deploy them on existing enterprise solutions.
- Makes informed decisions. At the core of generative AI is a multilayer neural network capable of processing vast amounts of data. Businesses can leverage the AI engine to support decision-making by reducing costly oversights.
- Personalized experience. Pre-trained generative AI models can be further fine-tuned with information on your products and services. This lets you engage customers by enhancing their journey with automated, relevant, and personalized responses.
- Virtual guidance. Generative AI opens up the possibility of smart AI trainers for the public. Businesses can train the model in various disciplines to help users explore new areas of interest.
- Content creation & inspiration. Language models like GPT are trained with large numbers of text. They can write poetries, creative stories, and quotes and perform other tasks to help content creators with imaginative works.
What are the drawbacks of generative AI?
While industries are raring to adopt generative AI, there are still concerns about its usage.
- Generative AI consists of large, complex models that are compute-intense to train and operate. For example, the GPT-4 is believed to have more than 1 trillion parameters. This makes the model extremely difficult and expensive to train. Moreover, pre-trained models require further fine-tuning with human feedback before they are fit for specific use cases.
- There are legitimate concerns that generative AI applications will replace the traditional workforce. Companies on a tight budget are already using AI to fill certain roles. Like all revolutionary technologies, generative AI will also create new opportunities, but the full implications remain to be seen.
- While AI can mimic humans, it might err when producing the output. We call this phenomenon 'bias', where the AI demonstrates prejudice or incorrectness from the data it was trained on. For example, an AI credit scoring system might reject a loan application simply because it does not have enough data on specific demographics.

What can Generative AI do?
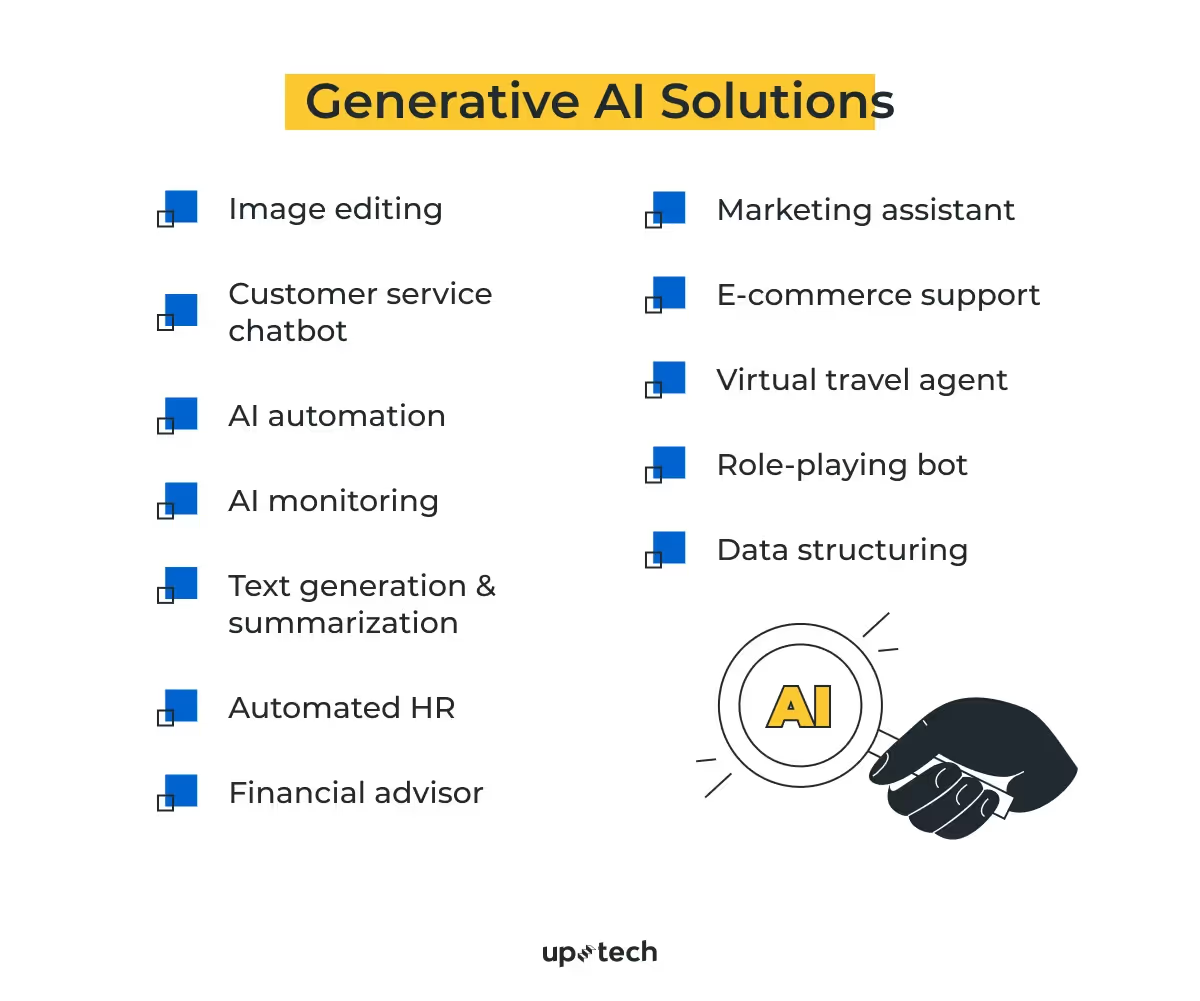
One of the most important tasks before building generative AI applications is understanding its capabilities. It helps you determine if it truly makes an impact on your operation and does not merely serve as a good-to-have accessory.
Here's a non-exhaustive list of practical generative AI applications in business.
Image editing
Images are helpful marketing elements in engaging customers and driving conversion. Image creation involves tedious design works until generative AI emerges. With AI design tools, users can automatically perform image retouch, upscaling, background removal, and other enhancements. For example, we built Dyvo, an image editor with AI capabilities, to allow users to generate unique avatars from selfies in seconds.
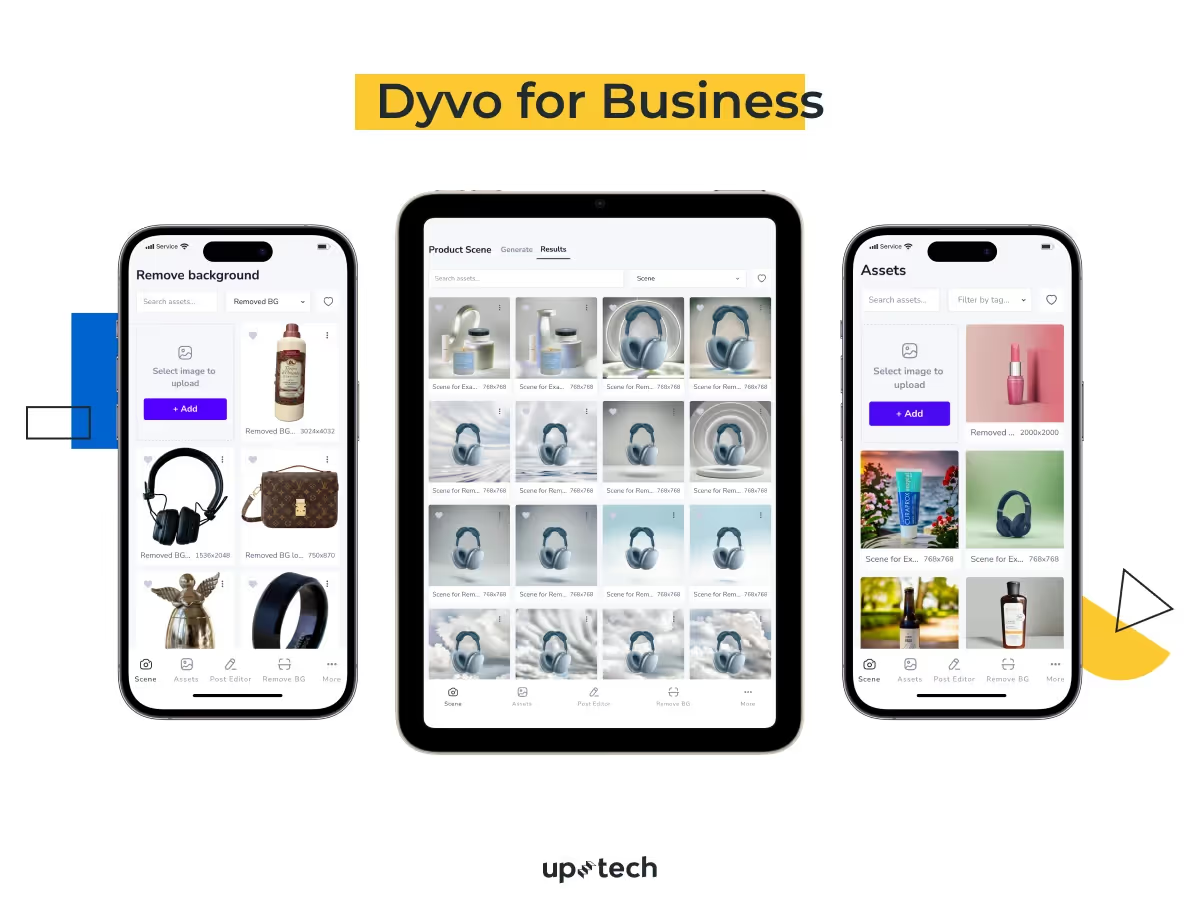
Customer service chatbot
Customer support teams are tasked to provide prompt and accurate responses to incoming queries and complaints. Scaling the support team proportionally to customer growth incurs substantial people and infrastructure costs. Instead, companies use generative AI technologies to build intelligent chatbots that can handle concurrent inquiries.
AI automation
Generative AI enables attended or semi-attended automation in various business workflows. For example, you can automatically compose an email by feeding the AI with previous customer replies. Besides being helpful with language, these AI models are also proficient in coding. This allows teams to automate code creation, API calls, and database management with ease.
AI monitoring
Generative AI can process large numbers of data in a very short moment and derive meaningful insights from them. This makes the technology helpful in performing active monitoring. For example, you can use generative AI to detect suspicious transactions or abnormal sensor data and raise alerts in real time.
Text generation & summarization

As linguistic models, generative AI can handle any language task. You can ask AI software like ChatGPT to summarize a lengthy text or explain complex concepts in simple words. AI writing software like Jasper also helps create various marketing copies, such as blogs, ads, and landing pages.
Check out our Hamlet case study.
Automated HR
Human resource management involves regular performance reviews, where managers provide employees with personalized recommendations and development plans. Now, generative AI is stepping up as a capable assistant for HR managers. For example, Plai, an online HR management solution, uses generative AI to provide recommendations and suggest follow-up actions based on individual feedback.
Financial advisor
A financial advisor's task involves predicting probable financial outcomes in the short, medium, and long term. When personalizing a financial plan, these professionals must consider various factors of their clients. Generative AI helps consolidate, analyze, and summarize information from multiple sources to assist financial planning.
Marketing assistant
Marketers will find an AI assistant helpful, particularly one capable of outlining ideas and performing basic research. Generative AI brings such capabilities to the fore. For example, marketers can use AI tools to research keywords, create social media strategies, or structure content for SEO purposes. Tools like Dyvo also allow marketers to create unique avatars in seconds, which helps them to engage their audience on various platforms.
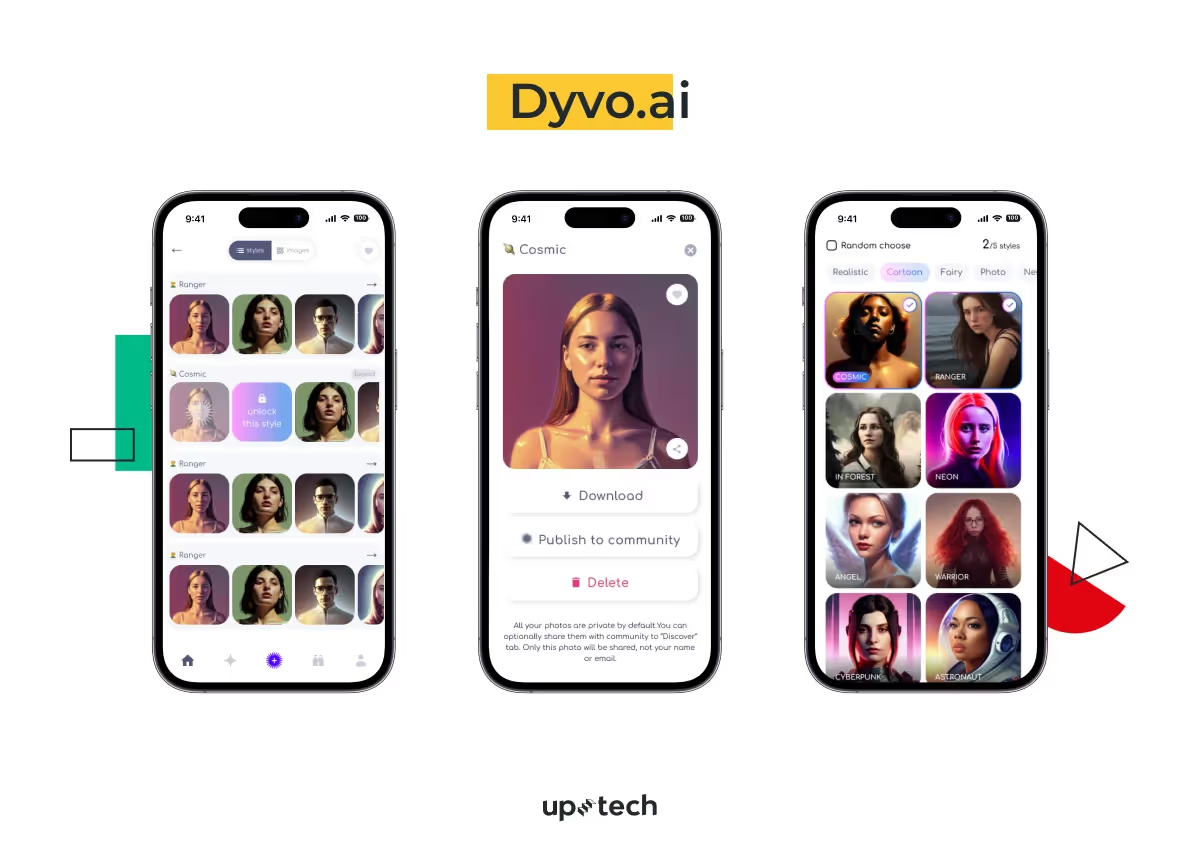
E-commerce support
Generative AI can be trained with the range of products an online retail store offers. When deployed as a chat agent, they can also style conversations with the preferred brand voice. This way, customers can initiate a request conversationally, such as 'Show me a dress for casual dining", and the chatbot will return the appropriate products.
Virtual travel agent
There's much to consider when planning a vacation, including the weather, flights, hotel, and places of interest. Instead of spending hours creating a travel plan, a generative AI application allows you to do so in minutes. It pulls data from the internet and creates an itinerary based on your travel purpose and preference.
Role-playing bot
Practice makes perfect, particularly if you're scheduled for an interview or going out on a date. Generative AI can take on a specific persona and interact with users like humans do. While it's not perfect, such applications allow you to anticipate, practice and respond to various scenarios before the event.
Data structuring
Generative AI might be in the spotlight for their text and image generation capabilities. But the technology is equally helpful in creating a data structure for technical applications. For example, data scientists can use ChatGPT to format information in JSON, Figma files, or instructions for specific machines.
How Generative AI is Changing Industries
The term generative AI might be new to many consumers, but the technology is already impacting various industries. Corporate leaders are using the deep learning model's capability to canvas large amounts of data and provide logical responses.
Fintech
Banks and alternative financial service providers leverage deep learning models like GAN for credit scoring and fraud detection. These models learn from vast financial data to pick up fraudulent transactions, spending patterns, and other credit factors. Then, these models are integrated into the bank's computer systems to automate and safeguard finance transactions.
Healthcare
In hospitals and medical establishments, generative AI strengthens efforts to improve patients' well-being in different ways. For example, generative learning models allow medical experts to recreate imaging data into realistic 3D forms. Such systems can also analyze patients' diagnoses, allowing medical workers to focus on delivering better care.
Real estate
Real estate agents seek better ways to market properties. They use generative AI solutions, such as Jasper, to create marketing copies. Some turn to AI visual design software to reproduce realistic property and interior design photos. There are also advanced AI software programs capable of producing a floor plan from textual description.
Ecommerce
Generative AI helps businesses improve ecommerce revenue growth in several ways. It helps generate appealing product descriptions and images for the online store. For example, marketers use Dyvo to create studio-quality product photos for ecommerce stores. Generative AI also enables online retailers like Amazon to generate personalized recommendations, which increases sales value per transaction.
Education
Generative AI changes how people learn and consume knowledge on the internet. Instead of subscribing to a fixed syllabus, they learn from personalized lessons that educators prepare with AI solutions. Moreover, AI allows teachers and coaches to assess students in real-time by analyzing assignments and summarizing the results.
Legal
Lawyers are burdened with paperwork and research and benefit from the robust capabilities of generative AI. Instead of searching for precedents or specific legal terms in archived documents, they can use AI to do the heavy lifting and concentrate on the creative parts of building the case.
Manufacturing
Manufacturers use generative AI systems to offer more predictability in logistics, market trends, and product demands. Advanced machine learning models can provide insights from real-time data to prepare manufacturers for changing market dynamics.
The Tech Stack we use to build Generative AI solution
Developing generative AI solutions requires mastering and integrating different machine learning and software development technologies. Our team has been actively following the trend in the AI space and adopted proven methods to bring advanced AI capabilities to our clients.
We use these technologies and frameworks to develop AI software from scratch.
- PyTorch and TensorFlow. Both are popular machine learning frameworks developers use to build and train machine learning models. We use these frameworks to debug and observe the model's behavior in different operating environments.
- Jupyter Notebook is an interactive platform our team uses to prototype AI applications. It consists of interactive features, allowing us to run and test small code units and get immediate results.
- HuggingFace provides open-source machine learning resources, such as pre-trained large language models and datasets for building AI software. It helps develop AI applications for natural language processing tasks like sentiment analysis and name entity recognition.
- Pandas is an open-source library by PyData that helps AI developers work with data structures. It comprises features that enable data manipulation, analysis, visualization, and more when building generative AI apps.
- OpenCV contains resources and libraries that help us build computer vision AI applications. For example, it provides functions enabling developers to implement object detection, feature extraction, and depth estimation.
- Scikit-learn is a Python-based framework that provides end-to-end machine learning development capability. It supports a wide range of machine learning tasks, such as regression, classification, and model selection.
- Cloud infrastructure and tools like AWS, Terraform, and GitHub allow us to build a gapless generative AI development workflow on the cloud.
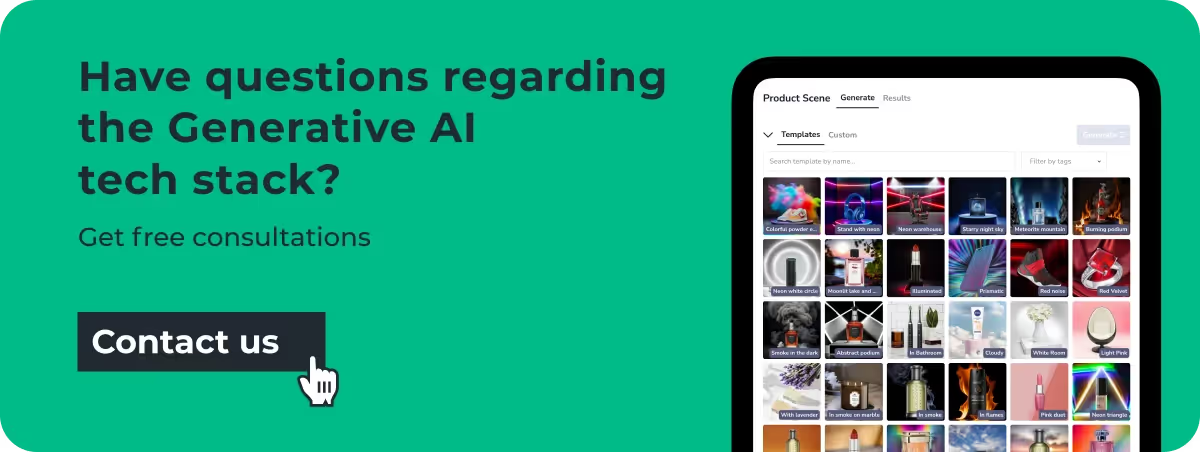
Step-by-Step Guide to Building Generative AI Solution
Like it or not, generative AI is here to stay and will disrupt various industries in the near future. However, you can make the technology works in your favor by building your own AI solutions. At Uptech, we've helped companies develop AI capabilities from scratch, and here's how we do it.
Step 1: Understand the problem
As powerful as they are, generative AI models are only purposeful when solving the right problem. Therefore, we'll first need to determine the exact problems you're trying to solve. For example, a public speaking training company might need an AI system to turn ideas into speeches. This differs from a video upscaler, which uses a computer vision model to reproduce old videos in high-quality formats.
Pro tips: Create a realistic performance baseline, which helps benchmark the model's actual performance when deployed. It allows you to manage expectations and suggest further improvements down the line.
Step 2: Select the appropriate model, algorithms, and tools
Depending on the application's purpose, we must assemble the relevant software tools, foundational model, and tech stack. For example, OpenCV is a helpful framework if we're building an imaging system that extracts and classifies objects from camera feeds. Then, we use platforms like Jupyter Notebook to enable seamless collaborations amongst data scientists, machine learning engineers, and developers. It's also helpful to set up a pipeline that allows developers to train, fine-tune, test, and deploy the model collaboratively.
Step 3: Gather and process data for training
Downloadable AI models from sites like Hugging Face must be trained with business-specific datasets before they are helpful for your apps. Here, we'll need to collect training data from different sources and clean and label them to train the model. For example, we perform tokenization and part-of-speech tagging when preparing textual training datasets. At this stage, our annotators work closely with domain experts to produce high-quality training data.
Step 4: Create a proof of concept
Start with a proof of concept rather than integrating a fully-trained model into a solution. Use a simple model capable of demonstrating basic capabilities to get feedback from the target audience. Note that the model may not produce the desired performance, but it allows you to test the idea and gather feedback from real users.
Step 5: Train the model (optional)
Next, we thoroughly train the model. We feed the AI model with the annotated datasets to learn specific patterns, which it later uses to solve business problems. Generative AI models use self-supervised and semi-supervised learning methods to train. Human involvement is essential to fine-tune and align the model's scope, accuracy, and consistency with the business objective. For that, we evaluate the model's performance with recall, F1 score, and other metrics.
However, this is a very expensive step. If you can apply existing models with minimal fine-tuning — it's usually a preferable approach.
Step 6: Integrate it into the application
Once we're satisfied with the model's performance, we apply the AI model to the application. This involves hours of coding, integration, and testing various app functions in different environments. When building the application, we consider scalability, data security, and error handling. Understandably, generative AI systems require immense computing power, and we might recommend shifting to GPU or TPU-based machines.
Step 7: Testing & adjusting parameters
A deep learning model consists of many hyperparameters, which impact its performance. For example, the number of layers, hidden unit, and dropout rate may cause overfitting or underfitting issues when misconfigured. Therefore, we run rigorous tests to ensure the model performs optimally. If needed, we apply hyperparameters optimization techniques such as random search and grid search to obtain the best performance.
Step 8: Publish
We then deploy the AI application on the public cloud or on-premise infrastructure. We practice DevOps culture and have a robust CI/CD pipeline to ensure you always have a production-ready build.
Step 9: Monitor & improve
Our team continues to provide post-release support and evaluate the model's performance in real-life use cases. We look for bottlenecks and instances where the model fails to analyze or produce relevant outputs with real-world data. Then, we run further tests, refine the model and revise the application. At the same time, our team studies user feedback to ensure the app has a good product-market fit.
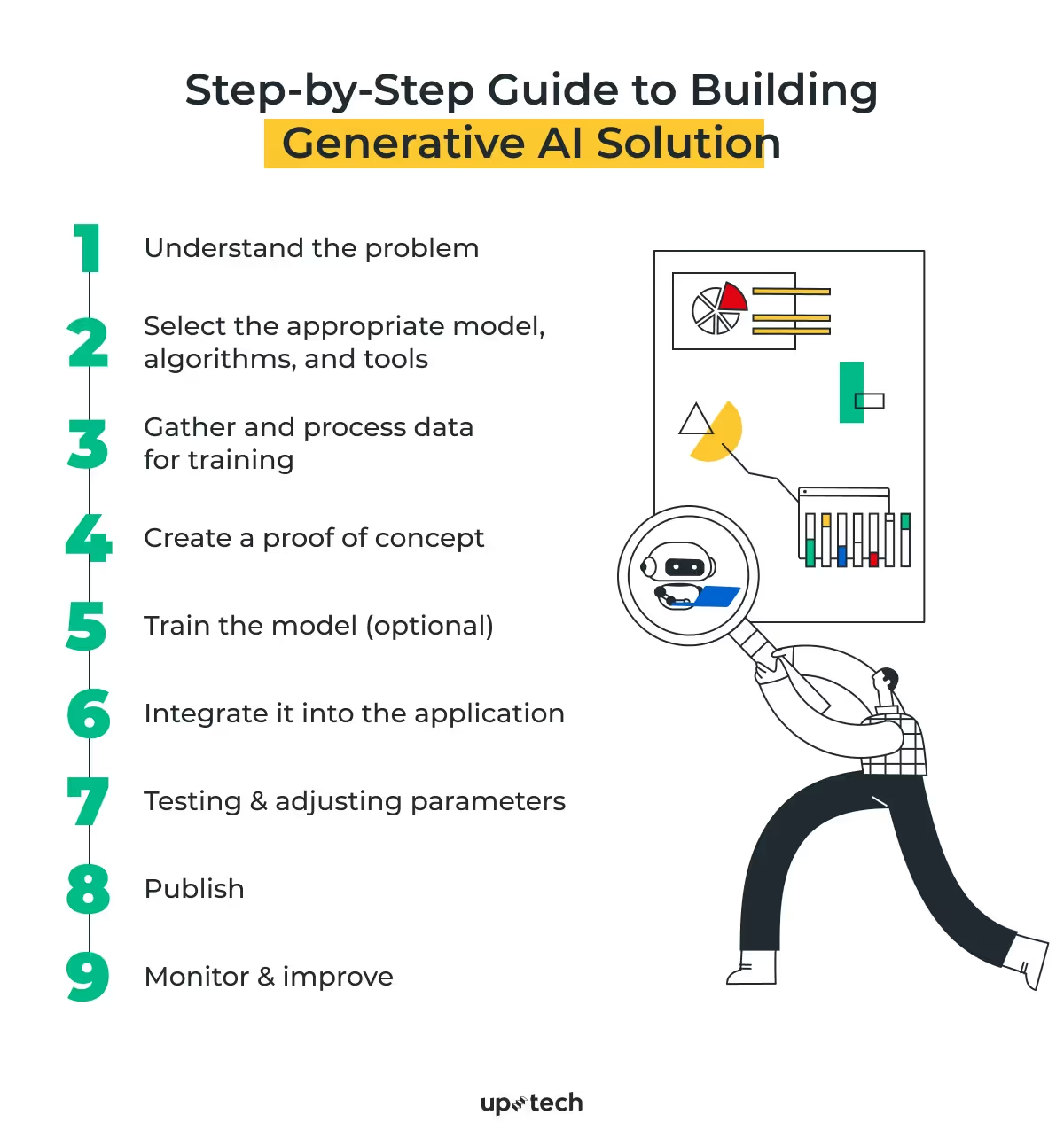
More Useful Tips for Building Generative AI Solutions
Learning how to build AI software gives you some clarity in the work involved. Still, there will be unanticipated risks and challenges that could jeopardize your efforts. Our team has gone on the path and picked up valuable experiences that might help.
Here are some helpful pointers.
- Identify specific parts of your business process or solutions that would benefit significantly from generative AI. Not all features need an upgrade, particularly with expensive AI technology. Instead, focus on changes that create the greatest impact for your users.
- Prioritize data protection to safeguard users' data and meet regulatory compliance. Training and operating generative AI involves moving large amounts of data around. Use protective measures, such as encryption, to reduce data risks.
- Generic AI software like ChatGPT might amaze the public, but they are difficult to use for specific business use cases. Furthermore, they are incredibly expensive to train. Hence, developing smaller but targeted AI models or fine-tuning pre-trained models make better sense.
- The training dataset's quality greatly influences model performance. Focus on preparing high-quality datasets to reduce performance issues like bias and under-generalization.
- Despite rigorous training, generative AI is not perfect. Therefore, it's important to state its limitations to your users. For example, OpenAI disclaims that ChatGPT may return incorrect and biased responses.

So, How Much Does it Cost to Build a Generative AI Solution?
The thought of developing generative AI software raises the question of affordability. Notably, training and deploying the model itself is a costly process. Add the standard development works, and you wonder if you might be staring at a hefty bill.
Yet, is AI development that expensive?
It depends on what you're trying to build and the solution's complexity. Unless you're making a generic AI tool like ChatGPT, you won't be paying astronomical sums to release an AI-powered app.
For context, our team can produce a PoC within 3 months while keeping the budget below $20,000. That said, remember that cost estimate depends on various factors, such as training requirements, app features, and the nature of the application.
Being in the app and AI development space for years, Uptech is known for striving to help startups and global companies adopt state-of-the-art technologies at a reasonable cost. We reduce risks and maximize investment by aligning each development stage with user feedback.
Summary
Generative AI has tremendous potential to revolutionize how businesses operate in various industries. It augments professionals and job roles with efficiency, automation, and fresh ideas. We've shown you how to make generative AI solutions and the tech stack we use at Uptech. Still, experience plays a crucial role in building functional AI solutions that satisfy users.
Talk to our team to learn more about turning your AI ideas into market-worthy solutions.