The healthcare industry is one of the early adopters of emerging technologies to improve patient care delivery. Generative AI (GenAI) is one such technology making its presence felt in the sector. When properly implemented, generative AI benefits wide-ranging healthcare services, including drug research, medical imaging, and personalized treatment.
According to McKinsey, proper use of generative AI can help companies tap into $1 trillion worth of opportunities in the medical industry. It allows doctors to gain valuable insights with generative AI models, while patients will be assured of the technology's efficiency and intelligence.
Yet, there are concerns that hospitals and medical institutions must address before they can implement generative AI at scale. If you’re a founder or product manager, exploring the subject matter in depth is sensible before building your own GenAI healthcare solution.
As the co-founder of Uptech, I’ve been closely following the developments in generative AI. We’ve also integrated the technology into several apps, including Dyvo, Plai, and Hamlet. Our work marked our understanding, skills, and business acumen in the respective industries.
In this article, I’ll share my thoughts about various generative AI use cases in healthcare, possible challenges, and best practices.
.webp)
How Generative AI will impact healthcare delivery
Generative AI is an advanced machine learning algorithm trained to produce new, unique content. It learns vast amounts of data to predict patterns and apply the stored knowledge to real-world information. For example, ChatGPT is a textual genAI tool capable of writing articles, while our product, Dyvo, uses generative AI to enhance product photos with artificially-generated backgrounds.
Generative AI is well-positioned to enhance healthcare delivery. Rather than deploying independently, GenAI integrates well with cloud infrastructure and IoT devices powering medical systems. Medical institutions generate and store large numbers of medical and patient data that AI models can process. By training machine learning to work on these data, you can create AI-powered systems that improve healthcare delivery on multiple fronts.
For example, medical staff and nurses are freed from repetitive administrative work as they can offload the burden of AI software. Meanwhile, doctors can speed up the time it takes to diagnose patients by integrating genAI into medical imaging systems. More importantly, patients benefit from the prompt and personalized treatment they can access remotely.
Overall, generative AI will enable healthcare institutions to
- reduce costs,
- mitigate risks,
- streamline operational workflow,
- and make better decisions when providing medical services
.
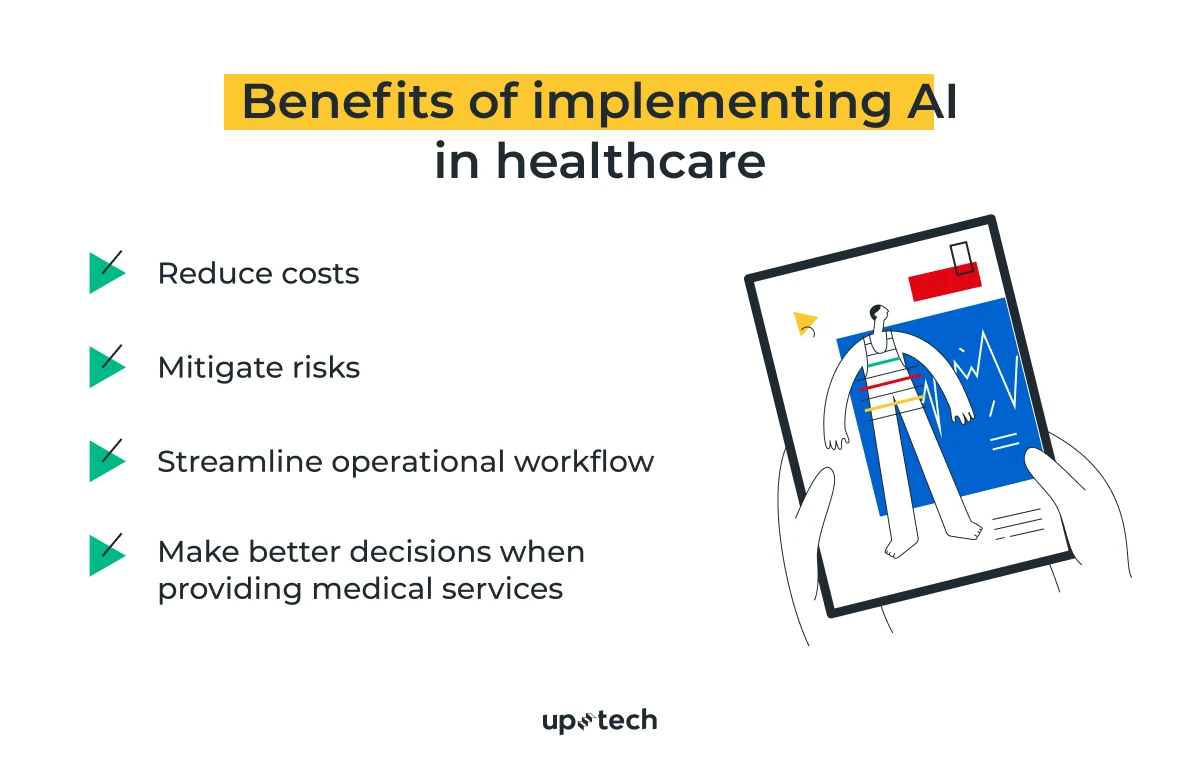
Practical use cases of Generative AI in healthcare
There are numerous ways to use GenerativeAI in medical facilities. I share several viable ones below.
Drug discovery
Research for new drugs requires medical scientists to canvas voluminous data for exploring new medicines and their potential side effects. Rather than undertaking the task manually, researchers can train generative AI models with existing drug datasets. Then the model can generate new drugs with unique molecular combinations and simulate their efficacy. Medical researchers would then review the results, approving or rejecting them accordingly. Nevertheless, deep learning models may reduce the time spent in early-stage testing, slightly reducing the drug’s time to market.
Medical chatbots
Generative AI consists of deep learning models capable of performing natural language tasks. When trained with medical data, the technology can power virtual assistants to assist patients seeking medical information. GenAI eliminates delays and provides coherent responses to casually-structured questions. For example, patients can report their symptoms to an AI medical chatbot, which furnishes them with pre-consultation advice.
Disease diagnosis
Generative AI can potentially enable timely intervention by spotting diseases in preliminary diagnoses. The deep learning model can analyze X-ray, MRI, and other medical imaging data to find similarities with patterns it has learned. Besides, AI can spot symptoms that may escape the clinician’s attention. This way, doctors can prescribe targeted treatment that might result in lesser complications.
Patient care
Doctors, clinicians, and medical staff can also use generative AI technologies as an assistant to support patient care. They can fine-tune the deep learning model with patient data, including previous medical histories. Then, the AI system can aid medical professionals by providing ongoing summaries of the patient’s condition. This allows doctors to focus on prescribing the appropriate treatment instead of being engaged with administrative work.

Research
Another example of generative AI in healthcare is its capability to support ongoing medical research. From analyzing volumes of medical literature to planning clinical trials, deep learning models allow researchers to be more efficient when advancing medical science. With generative AI, researchers can explore and validate new assumptions, potentially making key discoveries in lesser time.
Personalized treatment plans
Prescribing treatment to patients is challenging for physicians because of each individual's varying conditions. In this case, generative AI can analyze specific conditions and predict potential complications before suggesting a treatment plan. Then, physicians review the recommendations and treat the patients accordingly.
Predictive maintenance
Medical equipment is prone to wear and tear. If left unmitigated, equipment breakdown impacts hospital operations and patients’ well-being. To avert that, you can train generative AI to analyze the machine’s sensor data and predict the likelihood of failure. More importantly, genAI can suggest the type of intervention the machine needs to remain operational.
Medical robots
Generative AI enables the development of better-performing medical robots. Whether an assistive wearable device or remote-controlled surgical arms, these robotic gears require precise engineering. Generative AI can study existing models, identify strengths and weaknesses, and recommend improved versions.
Common challenges of Generative AI in Healthcare
Generative AI brings exciting opportunities for medical practitioners, patients, and service providers alike. Yet, industry leaders have been cautious when integrating technology with medical practices. If you’re developing GenAI products for healthcare applications, be wary of these challenges.
Training with the right dataset
Generative AI is only as accurate as the dataset it trains on. When developing GenAI systems for healthcare, it’s essential to train the model with high-quality medical datasets. This means collecting, cleaning, and labeling medical data such as imaging results, lab tests, and patient records with stringent guidelines.
Both data quantity and diversity are also crucial when preparing the training samples. Deep learning models need a sufficiently large amount of data to train on before they can generate new content accurately and consistently. Besides, the dataset adequately represents the domain where the generative AI operates. For example, if you’re training a medical chatbot, you must train it with patient data encompassing all demographic the hospital serves.
Applying the right deep learning algorithm
Generative AI typically uses neural network architecture like generative adversarial network (GAN), convolutional neural network (CNN), and transformer. Within those architectures, there are several variants with differing characteristics. Each deep learning model might perform differently in medical use cases. For example, CNN is suitable for developing imaging systems, but developers usually use transformers for language applications like medical chatbots.
Using generative AI ethically
The prospect of applying generative AI in healthcare also sparks ethical concerns. Deep learning models are prone to bias, particularly when it trains with datasets leaning towards a demographic or belief. A biased AI system exposes patients to inaccurate information that might jeopardize their well-being.
As helpful as they are, the generative AI model is meant to solve only some medical operational problems. For example, it is not optimized for arithmetic. Therefore, marketing generative AI for hospital billing systems introduces risks of inaccuracy in financial reports.
Managing data security and privacy
Training generative AI model requires storing and processing large numbers of sensitive medical data. Developers, service providers, and medical institutions must implement measures to safeguard patients’ privacy and comply with industry regulations. Otherwise, medical institutions risk data breaches and hefty penalties.
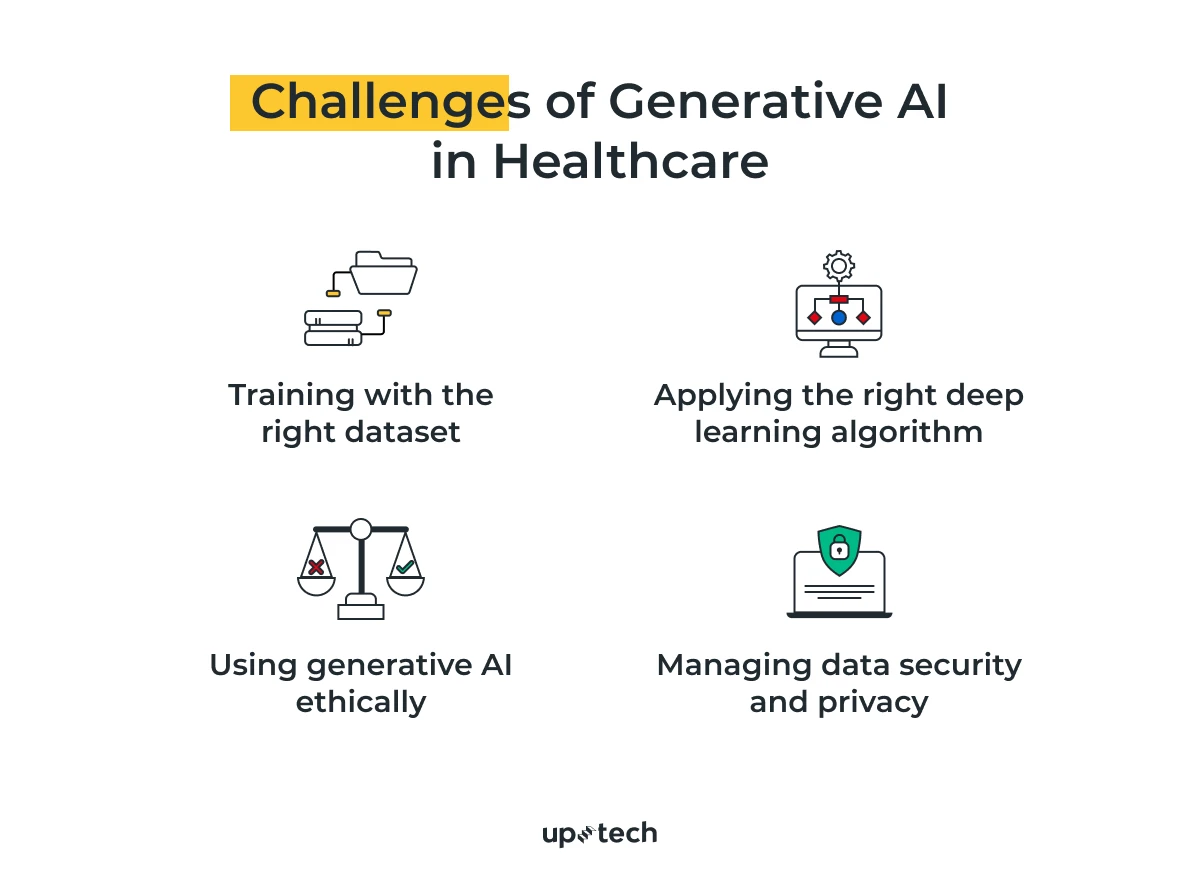
Helpful tips on using Generative AI in healthcare
Developing GenAI healthcare systems require a precise understanding of deep learning models and sensitivities surrounding the healthcare industry. Use these tips to ensure your healthcare generative AI product serves its purpose while providing data privacy.
- Protect training datasets you use for the models with ample security. For example, use HIPAA-compliant cloud infrastructure to train and deploy the machine learning model.
- Place equal importance on the quality of medical datasets for training the model. Carefully curate them from trusted sources and ensure they fairly represent the subject.
- If you use a deep learning model for calculations, be mindful of its limitation. Always employ a safeguard, such as an administrative officer, to review the generated results.
- Use pre-trained models instead training a model from scratch. Generic AI models like GPT cannot function in the medical context without fine-tuning. Some models might inherit more biases or inaccuracies from datasets.
- Continuously improve the model by training it with new data the system collects. This allows the model to refine its inference and provide more accurate results.
.webp)
Summary
Generative AI is poised to inject healthcare systems with efficiency and creative use cases. Doctors, nurses, and medical staff will benefit from the technology's intelligence and time-saving integrations. Likewise, patients will appreciate GenAI’s positive impact on medical research, disease diagnosis, patient care personalization, and other use cases.
Still, integrating genAI in a strictly-regulated industry is fraught with challenges. I’ve shared approaches to mitigate data risks, reduce costs, and maximize the model’s accuracy. Hopefully, they will prove helpful in your attempt to develop a functional genAI solution.
Alternatively, consult our AI experts to bring your ideas to life.