There’s been a lot of buzz around artificial intelligence lately – and not just in finance or logistics. AI in mental healthcare is drawing more and more attention, too. Can AI treat mental illness? Does it really help? Are there risks? Could it ever replace traditional therapy?
While it’s unlikely AI will replace therapists anytime soon, there's no doubt it’s already on the way to change how mental health support is delivered: The technology makes care more accessible, personalized, and proactive in the process.
I’m Oleh Komenchuk, ML Department Lead at Uptech. In this post, I’d like to share my thoughts on what’s driving the adoption of AI in mental health therapy, the benefits and challenges it brings, and how it’s being used today, from early diagnosis tools to personalized treatment apps.
Whether you run a mental health startup, lead a private practice, or manage a wellness platform, this guide will show you how to bring AI into your work in a meaningful way.
What Drives The Need for AI in Mental Health Therapy
This information won’t be brand new for you, probably, but I need to mention it. The global mental health crisis is here. And it is pushing healthcare providers, digital health startups, and therapists to explore smarter, more scalable ways of delivering care. AI is part of the conversation – not as a replacement for human support, but as a powerful tool to extend and improve it. So, what are the reasons behind the growing demand for AI in mental health diagnosis and therapy?

Growing prevalence of mental health conditions
Unfortunately, mental health disorders are becoming more common across all age groups. About 60 million Americans live with mental illnesses of different kinds and severity levels. Globally, at least 10% of the population is affected, and almost 15% of adolescents live with a mental health condition. Among people aged 15 to 29, suicide is already the fourth leading cause of death.
While increased awareness may be playing a role, the steady rise in diagnosed conditions signals a clear need for more accessible and responsive care.
Limited access to care
Despite rising awareness, access to mental healthcare remains far from universal. Studies report that only one in four Americans with a mental health condition can access care. The rest are left without any treatment, often due to high costs or a lack of local specialists. And in many countries, mental health services are underfunded and stretched thin.
Mental Health America’s 2023 report shows that nearly 30 million U.S. adults with mental health disorders don’t receive treatment – a gap that AI solutions can help bridge by supporting remote care, early screening, and patient monitoring.
Rising costs for patients and providers
Mental health issues aren’t just a public health concern – they also carry a huge economic burden. According to projections, mental illnesses will cost the global economy around $16 trillion between 2010 and 2030.
This pressure is felt on both sides: patients struggle with treatment costs, while clinics must balance limited budgets with growing demand. AI can relieve part of that load by automating tasks and enabling more efficient care delivery.
Demand for personalized, continuous care
Mental health is deeply individual. What works for one patient may not work for another, and that’s where AI steps in. Today’s users expect care that is not only accessible but tailored to their needs.
From adaptive treatment plans to intelligent progress tracking, AI helps build the kind of personalized, continuous care that today’s mental health landscape requires.
Global instability and chronic stressors
Wars, forced migration, economic recessions, and climate-related disasters all contribute to rising levels of stress, trauma, and uncertainty, often triggering or worsening mental health conditions. These large-scale stressors are difficult to address with traditional systems alone.
AI offers scalable tools that help assess risk early, support overwhelmed healthcare systems, and provide timely interventions even in unstable conditions.
The long-term impact of the pandemic
The COVID-19 pandemic didn’t just disrupt mental health services – it made mental health challenges more widespread and urgent. Lockdowns, social isolation, financial instability, and grief intensified psychological distress worldwide.
In the WHO European Region alone, over 150 million people were reported to be living with mental health conditions in 2021, with many struggling to access care.
The pandemic also accelerated the adoption of digital health tools, paving the way for more advanced AI-powered interventions.
Growing openness to digital tools in care
Over the past decade, people have grown more comfortable using digital tools for mental well-being, from meditation apps to virtual therapy sessions. This acceptance has laid the groundwork for more advanced AI-driven features to enter the picture.
When thoughtfully integrated, AI can improve the therapeutic process and make care more proactive, scalable, and responsive.
How exactly? You’re about to find out.
How AI Can Be Used to Support Mental Health Services
Mental healthcare has long been seen as a field only for humans, where empathy, emotional nuance, and human connection play a central role. Unlike radiology or oncology, where AI, computer vision in particular, already outperforms humans in some tasks, mental health poses a different challenge: how can a machine understand what someone feels?
That skepticism is real, especially among practitioners who view therapy as something deeply human. And yet, the reality is more nuanced. People often open up to AI, not because they expect deep emotional insight, but because they feel safe. Talking to a virtual assistant removes the fear of being judged. It’s anonymous, always available, and can be surprisingly comforting.
It can be something as simple as a check-in after a tough day or a guided therapy session through a chatbot. AI has started to prove its value, not as a replacement for therapists, but as a bridge to more consistent, responsive care.
The real question now is not if AI can support mental health, but how it can do so responsibly and effectively. I have rounded up a few use cases of AI for mental health.
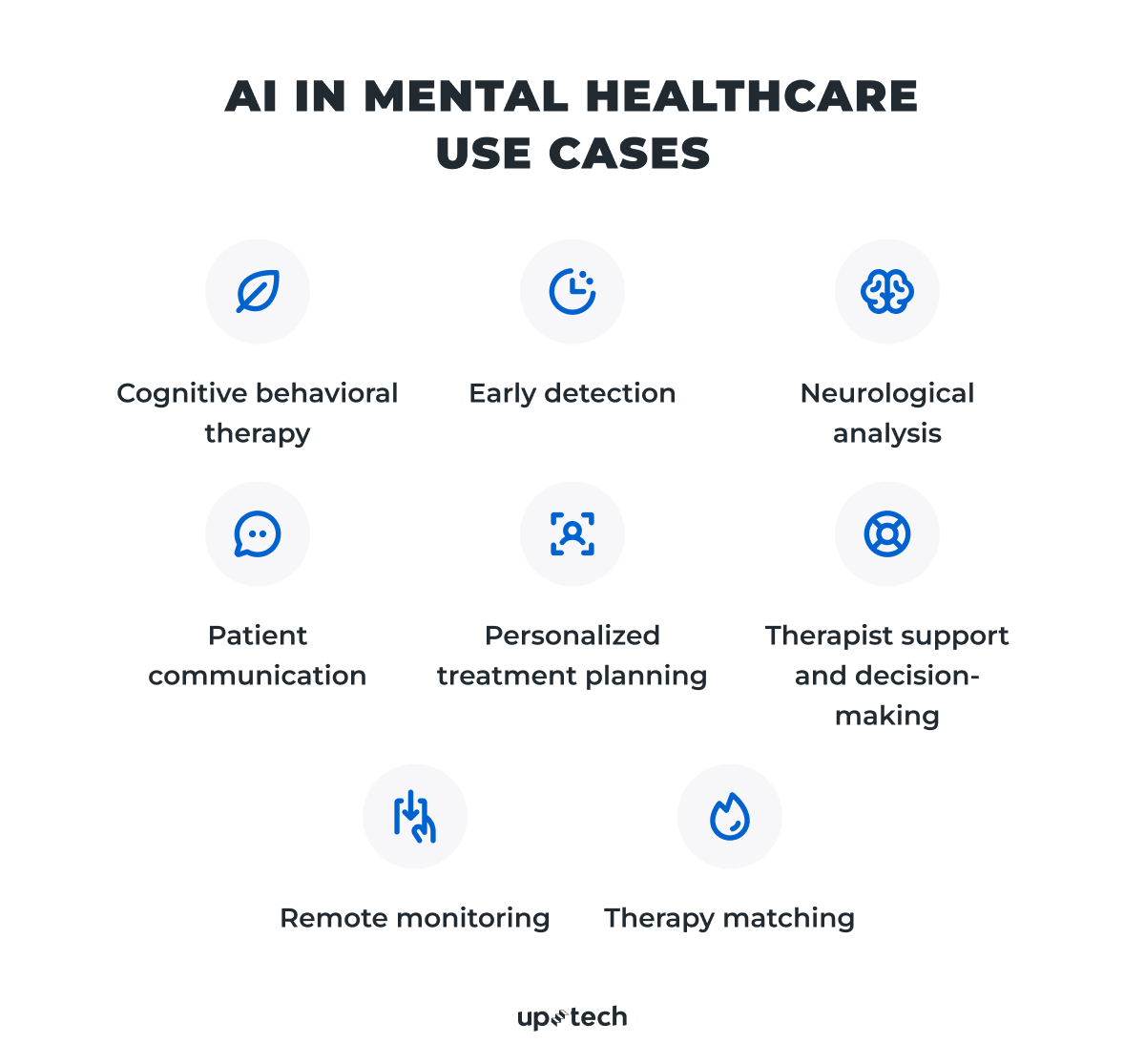
Cognitive behavioral therapy
Cognitive behavioral therapy (CBT) has proven effective for treating anxiety, depression, PTSD, and more. When it comes to AI in behavioral health, it brings CBT to mobile apps, chat platforms, and self-guided programs that are available 24/7. These tools walk users through therapeutic exercises, suggest coping strategies, and help track mood and behavior over time.
Many platforms now rely on natural language processing (NLP) to simulate therapist-like conversations. Some opt for generative AI services to tailor responses based on each user’s emotional state and therapy goals.
According to multiple meta-analyses, digital CBT can match, and sometimes surpass, traditional face-to-face therapy in effectiveness. That makes it a strong option for people who face cost, stigma, or access barriers.
And clinical regulators are taking notice. The FDA has cleared the first prescription digital therapeutic for major depressive disorder – a software app that helps modify behavior through structured lessons and exercises. That’s a major step toward recognizing tech-based therapy as a valid treatment option.
Early detection
AI helps clinicians catch mental health conditions at an early stage. AI models analyze patterns in speech, text, wearable data, and even social media activity, and can spot subtle shifts that suggest a person may be at risk.
Powered with machine learning (ML) algorithms, systems can detect indicators of depression, anxiety, or suicidal thoughts before a person seeks help. Some tools monitor sleep, screen time, or voice tone to flag warning signs. This kind of insight allows providers to step in early, adjust care plans, and reduce the chances of crisis escalation.
In one of the recent studies, machine learning models like support vector machines, decision trees, random forests, and logistic regression were used to predict relapses, hospitalizations, and suicide risk in people with bipolar disorder.
These models analyzed clinical records and brain scan data from over 30,000 patients and reached up to 98% accuracy in some cases. By picking up on early warning signs, like disrupted sleep, substance use, or changes in brain activity, AI gives providers a better shot at stepping in before things spiral.
Neurological analysis
AI brings value to the clinical side of mental healthcare, too. Especially when brain imaging is involved. In disorders like schizophrenia or ADHD, diagnosis often relies on pattern recognition across complex datasets. That’s where deep learning neural networks and computer vision come in.
AI systems process thousands of fMRI or EEG scans and learn to spot subtle markers of mental illness, sometimes before clinical symptoms appear. These tools enable researchers to identify neurological patterns and also give clinicians a second opinion to support diagnosis.
AI also helps monitor how patients respond to medication or therapy via the analysis of brain signals.
Patient communication
AI tools now make it easier for patients to stay engaged and feel supported, even outside scheduled therapy. Conversational agents, or mental health chatbots, rely on NLP to hold context-aware dialogues, ask emotionally intelligent questions, and offer psychological first aid.
For many users, talking to AI feels less intimidating than opening up to a human. As mentioned earlier, it removes the fear of being judged and offers instant, always-on access. Some platforms combine speech recognition with emotion analysis to assess tone and detect distress in real time. Such a system may prompt follow-up questions or encourage the user to contact a human professional.
Personalized treatment planning
AI also helps tailor treatment plans based on individual needs. They do not just assign a generic protocol. Instead, systems analyze patient history, symptoms, progress, and even genetic data to suggest therapy formats, durations, or medication combinations that are more likely to work.
Reinforcement learning models can be used here to continuously adapt recommendations based on how a person responds. The result? The therapy is tuned into a dynamic, personalized experience. For therapists, this means more accurate treatment decisions. For patients, it can mean faster relief.
Therapist support and decision-making
AI doesn’t just assist patients; it supports clinicians, too. Some tools, powered with speech recognition technology, help transcribe and summarize therapy sessions, detect patterns across a therapist’s caseload, or flag high-risk cases based on symptom changes.
Intelligent document processing tools pull insights from clinical documentation using NLP, helping therapists prepare for sessions more efficiently. This reduces cognitive load and leaves more time for meaningful interaction. In team settings, AI can also surface trends to inform supervision and planning.
Remote monitoring
Mental health doesn’t follow a schedule, and many patients experience fluctuations between sessions. That’s where AI remote monitoring steps in. Such systems collect and analyze data from wearables, mobile apps, and user behavior, and can help track changes in mood, energy levels, sleep, and more.
For example, if a user’s app activity drops or their sleep becomes irregular, ML models can flag these shifts as potential signs of relapse or emotional decline. Some systems go further: They use speech analysis or facial recognition to detect stress, fatigue, or agitation during video calls.
Remote monitoring keeps care continuous. It allows providers to respond faster, personalize interventions, and check in with patients before small issues spiral into crises. It’s especially useful in outpatient care, long-term treatment, and rural or underserved communities.
Therapy matching
Not every patient connects with the first therapist they meet, and mismatched care often leads to dropout. AI can analyze factors like patient needs, communication preferences, therapy history, and even linguistic style to recommend a therapy approach that best fits a specific person.
Some systems use LLMs, NLP, and collaborative filtering to match individuals with therapists who are more likely to align with their expectations and communication styles. Other factors include specialty areas, cultural background, or availability to recommend a best-fit provider.
To learn how collaborative filtering works, read our article about recommendation systems.
Better matches from the start improve engagement, trust, and outcomes. This use case is especially valuable for digital mental health platforms or clinics with large therapist networks.
We at Uptech developed an internal mental health platform that uses AI to match users with certified CBT therapists based on their goals, availability, and personal preferences. The platform includes a dynamic questionnaire, intelligent scheduling suggestions, and a built-in chat for direct communication before the first session. By helping users find their ideal therapist more efficiently, our solution improves the overall experience for both patients and providers. It demonstrates how AI can solve real-world problems in mental healthcare.

Challenges to Incorporating Artificial Intelligence in the Mental Health Sphere
AI has a lot to offer in mental healthcare, but it’s not a magic wand. Like any tool, it comes with limitations that developers, clinicians, and decision-makers need to address. That doesn’t mean avoiding AI altogether. It means taking a thoughtful, responsible approach that turns these challenges into opportunities for better, safer care.
Here are some of the most common concerns, and what can be done about them.
Narrow focus of current research
Most existing studies concerning AI in mental healthcare focus on just a few conditions, like depression and schizophrenia. That leaves out many other issues, such as anxiety disorders, eating disorders, or trauma-related conditions.
Solution: Future AI systems should be trained on broader, more inclusive datasets. Companies and researchers can also work together to test tools in underrepresented areas – whether that’s adolescent mental health, postpartum depression, or chronic stress.
Risk of bias and inaccurate predictions
AI models are only as good as the data they learn from. If that data is limited, messy, or unbalanced, the results can be misleading – or worse, reinforce existing biases in mental healthcare. Poor validation, rushed development, or overfitting can also make models look more accurate than they actually are.
Solution: The key is careful, transparent development. It is important to have enough high-quality data and models that are trained on real-world data. Specialists may apply techniques like cross-validation and work with diverse datasets to minimize bias. It also helps to involve mental health professionals throughout the process, not just at the end.
Lack of transparency and replicability
Many AI models are built in black boxes. It’s often unclear how they work, how they were trained, or why they make certain predictions. That creates trust issues, especially in a field as personal and sensitive as mental health. It also makes it hard for other developers or clinicians to build on existing work.
Solution: Teams building mental health AI apps and tools should document their approach clearly and make models as explainable as possible. Explainable AI (XAI) techniques are one of the most effective ways to do that. XAI shows which features influenced a prediction or highlights the model’s decision-making path. It also helps bridge the gap between black-box systems and human understanding, making it easier for both clinicians and users to trust the output.
Open-sourcing parts of the code or datasets, where privacy allows, can also help foster transparency and collaboration across the industry.
Poor data management and preparation
Behind every AI system is a lot of data, like really a lot of data. But in many mental health projects, that data is underprepared or misunderstood. Problems like missing values, inconsistent labeling, or mismatched formats can seriously affect a model’s performance. On top of that, privacy and consent are huge concerns when handling sensitive mental health data.
Solution: Strong data engineering should be a core part of any AI project. That means careful preprocessing, secure storage, and strict privacy practices. When you work with healthcare data, you need clear consent protocols and compliance with regulations like HIPAA or GDPR.
Hype without real-world testing
It’s easy to get excited about what AI might do. But in practice, some tools are pushed out before they’re ready, with little testing in real-world clinical environments. That creates unrealistic expectations and can stall adoption altogether.
Solution: AI models should be developed with practical use in mind. It’s super important to test mental health AI systems in real clinical workflows, not just academic environments. Collaboration between developers, therapists, and product teams helps create tools that actually support care, rather than distract from it.
Uptech Can Help You Build A Robust Mental Health App
Traditional therapy, as human and compassionate as it is, often ends up relying on general assumptions. That’s because therapists work with limited time, stretched resources, and diagnoses that can be highly subjective.
AI, on the other hand, brings data into the picture. It can analyze thousands of cases, spot patterns that aren’t obvious to the human eye, and support therapists with objective insights.
At Uptech, we’ve seen firsthand how AI can create real value in healthcare, not just in theory, but in practice. Here are a couple of examples where we helped our clients turn complex challenges into tangible results:
We helped a private diagnostic clinic build an AI-powered medical image processing system to speed up diagnostics and reduce human error. Using models like U-Net, DeepLab, and EfficientNet, the platform now automates image classification, segmentation, and anonymization, cutting analysis time by up to 30%. The solution also meets strict data privacy standards, including HIPAA compliance, and supports both 2D and 3D imaging.
We partnered with a private U.S. clinic to build an AI-powered medical document processing system that automates classification, search, anomaly detection, and communication. Using technologies like OCR, BERT-based NLP, and anomaly detection models, the platform reduced document processing time by up to 30% while improving data accuracy and patient satisfaction. The system now helps healthcare staff spend less time on paperwork and more time delivering care.
These projects show what’s possible when AI meets the real needs of patients and healthcare teams, with the right technology, the right expertise, and the right mindset.
Curious about how AI can support your mental health platform or clinic? Let’s talk. Our team at Uptech is ready to help you bring smart, responsible AI solutions to life.
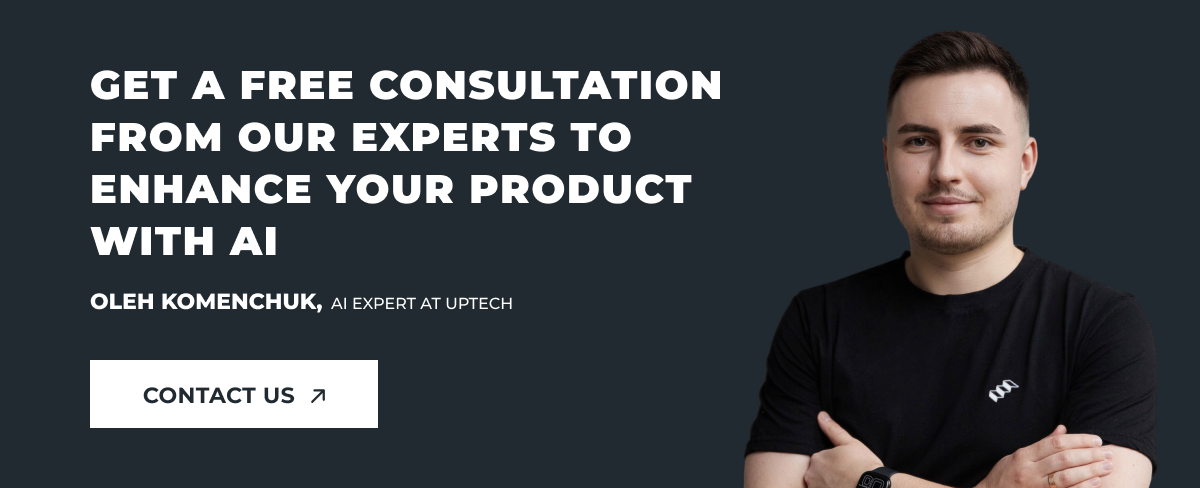